Yanglong LU 陸楊龍
Yanglong LU 陸楊龍 Details
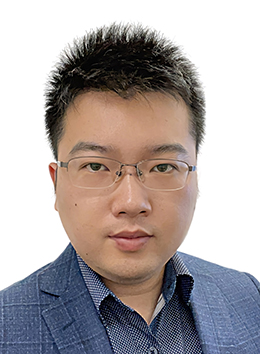
Contact Information
Research Interests
Biography
Prof. Lu received his Ph.D. and B.S. degrees both in the Department of Mechanical Engineering at Georgia Institute of Technology. He received ASME Computers and Information in Engineering Division (CIE) Best Ph.D. Dissertation Award in 2021. Before joining HKUST as an assistant professor in 2022, he worked as a postdoctoral research fellow at University of Michigan.
Prof. Lu’s research interests are process modeling and monitoring in additive manufacturing, design optimization, and human health monitoring and diagnosis. Variability of build qualities is one of the major challenges in additive manufacturing. Our group develops novel sensing techniques to improve efficiency and accuracy in process monitoring by integrating physical models and machine learning methods. Physics-informed machine learning also provides a new direction for human health monitoring, where available data are very limited. Our group also designs metamaterial with novel properties by structural optimization methods.
Research Interests
Biography
Prof. Lu received his Ph.D. and B.S. degrees both in the Department of Mechanical Engineering at Georgia Institute of Technology. He received ASME Computers and Information in Engineering Division (CIE) Best Ph.D. Dissertation Award in 2021. Before joining HKUST as an assistant professor in 2022, he worked as a postdoctoral research fellow at University of Michigan.
Prof. Lu’s research interests are process modeling and monitoring in additive manufacturing, design optimization, and human health monitoring and diagnosis. Variability of build qualities is one of the major challenges in additive manufacturing. Our group develops novel sensing techniques to improve efficiency and accuracy in process monitoring by integrating physical models and machine learning methods. Physics-informed machine learning also provides a new direction for human health monitoring, where available data are very limited. Our group also designs metamaterial with novel properties by structural optimization methods.